Our world is built on biology and once we begin to understand it, it then becomes a technology.
– Ryan Bethencourt
ABSTRACT
American scientist Jennifer A. Doudna and French scientist Emmanuelle Charpentier (sixth and seventh women Nobel prize winners) co-invented CRISPR/Cas9 gene editing. CRISPR stands for Clustered Regularly Interspaced Short Palindromic Repeats. It is a piece of genetic information that some bacterial species use as part of an antiviral mechanism.
CRISPR-Cas9 has a strong relationship with bioinformatics. CRISPR-Cas9 is a genome editing tool that allows researchers to make precise changes to DNA sequences. To use this tool effectively, researchers need to design guide RNAs that can recognize and target specific DNA sequences. Bioinformatics tools and techniques are essential for designing these guide RNAs and analysing the data generated by CRISPR-Cas9 experiments.
HOW DOES CRISPR CAS 9 WORK:
CRISPR is a natural process that functions as a bacterial immune system against invading viruses. They use two main components. The first is short snippets of repetitive DNA sequences called CRISPR. The second is Cas or Crispr-associated proteins which chop up DNA like molecular scissors.

When a virus enters a bacteria Cas proteins cut out a segment of the viral DNA to stitch into the bacterium’s CRISPR region, identifying the chemical code of the infection. Those viral codes are then copied into short pieces of RNA. This RNA binds to a special protein called Cas9. The resulting complex acts like guards latching onto free-floating genetic material and searching for the match to the virus. If the virus invades again, the complex recognises it immediately and Cas9 destroys the viral DNA. Lots of bacteria like halophiles, E. coli, and clostridium have this type of defence mechanism.
CRISPR CAS9 IN MICE:
The CRISPR/Cas9 system simplifies the entire process of creating knockout mouse models. This technology is used to knock out or modify DNA in research mice to study disease phenotypes and develop new treatments. A knockout mouse is a genetically modified mouse in which researchers have inactivated, or “knocked out”, an existing gene by replacing it or disrupting it with an artificial piece of DNA. Using CRISPR we can mutate several suspected cancer genes simultaneously in the somatic cells of adult mice.
CRISPR knock-ins have also corrected disease-causing gene defects in adult mice, such as the mutations that cause haemophilia and sickle cell anaemia. CRISPR was previously used to treat progeria and muscular dystrophy in mice.

PROGERIA IN MICE
DISEASES THAT CAN BE CURED USING CRISPR CAS9
CRISPR technology could cure many human hereditary diseases such as haemophilia, β-thalassemia, cystic fibrosis, Alzheimer’s, Huntington’s, Parkinson’s, tyrosinemia, Duchenne muscular dystrophy, Tay-Sachs, and fragile X syndrome disorders. CRISPR is used to edit the gene by changing the DNA from a harmful variant to a healthy variant. In addition, CRISPR is now being developed as a rapid diagnostic.
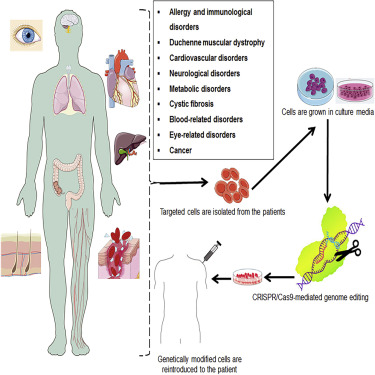
CRISPR CAS9 BIOINFORMATICS
In recent years, the CRISPR-Cas system has also been engineered to facilitate target gene editing in eukaryotic genomes. Bioinformatics played an essential role in the detection and analysis of CRISPR systems and here we review the bioinformatics-based efforts that pushed the field of CRISPR-Cas research further. CRISPR-Cas design tools are computer software platforms and bioinformatics tools used to facilitate the design of guide RNAs (gRNAs) for use with the CRISPR/Cas gene editing system. The performance of CRISPR/Cas relies on well-designed single-guide RNA (sgRNA), so a lot of bioinformatic tools have been developed to assist the sgRNA. These tools vary in design specifications, parameters, genomes etc.
Bioinformatics can help identify potential off-target effects of CRISPR-Cas9, which is critical for ensuring the specificity of the tool. Additionally, bioinformatics can be used to analyse the genomic data generated by CRISPR-Cas9 experiments to identify the effects of specific genetic modifications.
CONCLUSION
Compared with traditional gene-editing technology, CRISPR-Cas9 has a higher gene-editing efficiency, lower off-target effect, and no DNA integration, so it is an ideal gene-editing technology. They can modify DNA with great precision. This technique is known for its simplicity and efficiency. It also reduces the time required for the modification of target DNA.
Overall, the use of CRISPR-Cas9 in research is highly dependent on bioinformatics to design guide RNAs, analyse data, and ensure the specificity and safety of the tool.
Written by: Sathiga Devi P, 1st-year B.Tech Biotechnology